Writing a Generative AI Usage Policy - Ethical Considerations and Implementation Guidelines
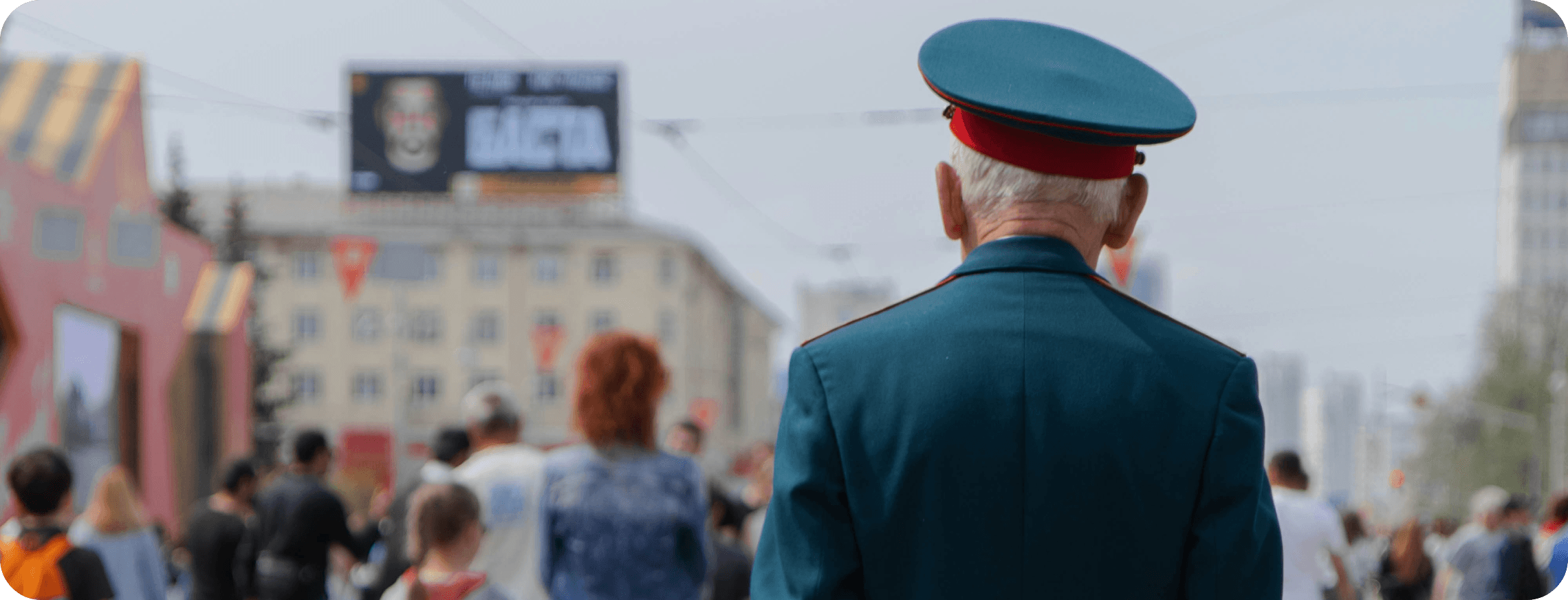
Background
Generative AI, a subset of Artificial Intelligence, has become an influential tool in the creation of original content. Unlike traditional AI, which primarily focuses on analyzing and recognizing existing data, Generative AI takes a step further by leveraging its learning from extensive datasets to produce entirely new images, music, text, and even code [1] [2]. This advancement not only demonstrates the innovative capabilities of AI but also highlights a growing need to address ethical considerations such as originality, copyright, and the potential misuse of generated materials [3].
The burgeoning potential applications of Generative AI are vast, spanning numerous sectors including drug discovery, the creative industries, and beyond [2]. A recent study projects that the Generative AI market will soar to an estimated value of 1.3 trillion dollars by 2032, indicating its transformative impact on various industries [3]. However, with such potent capabilities, the ethical development and deployment of Generative AI are of paramount importance to prevent issues like bias and misuse.
The data that Generative AI systems learn from significantly influences their outputs. If this data is skewed or imbalanced, it can result in biased outcomes, posing serious implications in real-world applications [3]. For example, AI-driven recruitment processes that inadvertently favor certain candidates over others due to biased training data can lead to unfair disadvantages, while AI-generated news articles with inherent biases may propagate misinformation and societal divisions [3].
Developers are therefore encouraged to implement strategies for mitigating bias, such as carefully curating training data and applying debiasing techniques, to ensure more ethical and responsible AI systems [4]. These efforts contribute to the creation of a future where Generative AI technology is leveraged responsibly and ethically for the benefit of all [4].
Key Components of Usage Policy
In drafting a usage policy for generative AI in legal settings, it is essential to address both the risks and ethical challenges that come with integrating AI into legal practices. Given the rapid evolution of this technology, any policy must be adaptable and comprehensive to ensure legal professionals remain compliant with their ethical and legal responsibilities.
Ethical Considerations
The policy should explicitly outline ethical considerations, emphasizing the need for human oversight. Generative AI cannot replace human expertise, and legal professionals remain accountable for AI's use in their work [5]. It is critical to highlight the importance of protecting client confidentiality, stressing that any use of AI that inadvertently shares confidential client information without informed consent is an ethical violation [5].
Understanding and Training
The policy should mandate thorough training for all users to understand the technology's capabilities and limitations. Legal professionals have an ethical obligation to comprehend the technology they employ, in line with the duty of providing competent representation as outlined in ABA Model Rule 1.1. This includes staying informed about the AI platform's training, limitations, and appropriate uses [5].
Risk Mitigation
Addressing the risks of AI usage is crucial. The policy must include guidelines on minimizing both output and input risks. Output risks involve the potential for AI to provide incorrect information confidently, known as hallucinations, which is particularly concerning given the current scarcity of accurate legal data in LLMs [5]. The policy should suggest strategies like incorporating more legal-specific data to train LLMs for increased accuracy. Input risks, primarily concerning breaches of confidentiality, should also be addressed by ensuring that AI platforms do not retain sensitive data or allow third-party access [5].
Procedural and Substantive Issues
The usage policy should anticipate and prepare for procedural and substantive legal issues that may arise. This includes addressing potential challenges to evidence admissibility generated by AI, and ensuring compliance with industry-specific regulations, especially in sectors like banking and finance where AI's informational basis may not be transparent to regulators [5]. The policy should also consider potential litigation risks, including legal malpractice and copyright claims resulting from AI use [5].
Licensing and Agreements
To safeguard confidential information, the policy should advocate for the signing of licensing agreements with AI providers. These agreements should include stringent confidentiality provisions to prevent unauthorized data retention or access [5]. This is crucial even when using platforms that have begun implementing privacy functionalities like disabling chat histories.
Continuous Evaluation and Updates
Finally, given the dynamic nature of generative AI, the policy should encourage continuous evaluation and updates. Legal professionals should regularly review and revise the policy to keep pace with technological advancements and emerging legal standards, ensuring ongoing compliance and risk mitigation [5].
Guidelines for Implementation
The implementation of a Generative AI Usage Policy requires both individual and organizational efforts to ensure ethical and effective use of generative AI technologies. At the individual level, researchers and creators must engage in due diligence by thoroughly investigating the capabilities, limitations, and terms of service of generative AI tools before choosing to utilize them [6]. It is critical for individuals to align their choices with personal ethical standards, much like selecting fair trade or sustainably made products. Particular attention should be given to terms of service related to copyright, privacy, data ownership rights, and data usage by third-party providers. If terms appear unclear or exploitative, they should be rejected. Many providers offer flexible options to opt out of data sharing and tracking history, which should be utilized to protect privacy [6].
For those disseminating work, it is essential to respect the publication or exhibition venue's accepted practices. This includes adhering to specific journal guidelines regarding AI-generated content, such as those from Springer Nature, which require that Large Language Models (LLMs) be documented in the Methods section and restrict AI-generated images unless sourced legally [6]. Similar policies exist across various academic publishers, requiring appropriate disclosure of AI usage in the research process, thus ensuring honesty, traceability, and accountability [6].
Furthermore, researchers should balance the use of generative AI tools with their own responsibility, verifying the accuracy and quality of AI-generated outputs and safeguarding sensitive data from bias or misrepresentation [6]. Disclosure of generative AI usage in research should be comprehensive, including a summary of the tasks performed with AI, tool citations, usage timestamps, prompts given to the tools, and archiving of unedited outputs for full traceability [6].
At an organizational level, institutions like the University of Illinois Urbana-Champaign provide a framework to support individuals by offering consistent guidelines that alleviate administrative burdens [6]. The institution ensures that its approved AI vendors comply with privacy and acceptable use policies, offering resources such as training and infrastructure to facilitate responsible AI usage. By providing these resources, the institution supports its members in adhering to both legal obligations and best practices in AI deployment [6].
Collectively, these guidelines for implementation serve to foster an environment of responsible AI usage, ensuring that both individual and institutional practices uphold the principles of integrity, transparency, and ethical conduct in research and publication [6].
Techniques for Bias Mitigation
In the realm of generative AI, addressing bias is crucial to ensuring fair and equitable outcomes. Bias in AI can manifest in various forms, such as word embedding bias, sample bias, and algorithm bias, among others [7]. To mitigate these biases, several strategies can be employed across different stages of AI model development.
Pre-Training Techniques
Pre-training bias mitigation involves preparing the dataset before it is used to train AI models. One innovative method in this stage is the creation of a mitigated bias dataset through a mitigated causal model. This approach adjusts cause-and-effect relationships and probabilities within a Bayesian network to ensure fairness [8]. Additionally, diverse team involvement during this stage is pivotal. A diverse team can enhance the representation in datasets and help identify potential biases that a homogenous team might overlook [7].
Training Techniques
During the training phase, algorithms should be equipped with bias detection and correction capabilities. A novel mitigation training algorithm for causal models can be implemented to address and reduce biases actively as they arise [8]. Human oversight, or having humans-in-the-loop, is another essential strategy. This approach allows for the real-time identification and rectification of unintended biases, leading to a more balanced model [7].
Post-Training Techniques
Post-training strategies focus on evaluating and refining AI models after their initial development. Developing interactive demonstrations to display experimental results can help understand and explain biases present in models. This transparency enables developers to replicate and refine the work to improve bias mitigation [8]. Open-sourcing models, as practiced by companies like StabilityAI, encourages community collaboration to enhance bias evaluation techniques and foster the development of solutions beyond basic prompt modification [9].
Maintaining Sensitive Features
Throughout the AI development process, it is critical to maintain and monitor sensitive features in the dataset to ensure that vital attributes are not overlooked or mishandled. This careful maintenance can prevent the exclusion of important data points that might otherwise lead to exclusion bias [8]. Documentation of data selection methods and cleansing processes is essential to track potential biases and mitigate their root causes [7].
By integrating these comprehensive strategies across the lifecycle of AI development, organizations can work towards minimizing bias and fostering more equitable generative AI systems.
Case Studies
The use of generative AI in various industries presents both innovative applications and challenges related to legal, ethical, and social implications. Below are several case studies illustrating the diverse uses and impacts of generative AI across different sectors.
Mastercard
Mastercard has effectively integrated generative AI into its marketing strategies through its proprietary Digital Engine. This technology analyzes billions of online conversations in real time to identify emerging micro-trends relevant to Mastercard's interests, such as travel and entertainment. Upon identifying a relevant trend before it peaks, the marketing team is alerted and can strategically engage with tailored social media posts and targeted ads. A campaign in collaboration with a national airline to promote a local tourist destination demonstrated the efficacy of this approach, resulting in a 37% increase in click-through rates and a 43% boost in engagement, while costs per click and engagement decreased by 29% and 32%, respectively [10].
Under Armour
Under Armour has utilized AI in its retail operations to enhance customer experience. Through a partnership with FitTech, customers can scan their feet in-store to receive personalized footwear recommendations. This integration of AI not only helps customers make informed purchase decisions but also streamlines the shopping process. Additionally, Under Armour leveraged generative AI for creative marketing efforts by using ChatGPT to generate scripts for its advertising campaigns, as seen in their revival of the "Protect This House" campaign [10].
Spotify
Spotify employs generative AI to personalize customer experiences and maintain its competitive edge. The company's predictive algorithms map customer journeys from initial interaction, tailoring recommendations to enhance user engagement. This personalized approach has contributed to Spotify's success, with 226 million users subscribing to Spotify Premium. Spotify's use of AI extends beyond its well-known AI DJ feature, encompassing refined data models that adapt to changing business needs [10].
easyJet
In the airline industry, easyJet has adopted conversational AI technologies to improve customer interactions. The company's Speak Now feature allows users to interact with a voice-assisted interface integrated into their mobile app, delivering information seamlessly through voice commands. This use of AI has been further enhanced with a chatbot boasting a 99.8% accuracy rate across 5 million queries, ensuring efficient and effective customer service [10].
Netflix
Netflix continues to leverage generative AI for content production decisions. By building models from users' data, Netflix analyzes attributes of past projects to inform the development and promotion of new content. This data-driven approach remains central to Netflix's strategy for producing exclusive titles that drive subscription growth. The ongoing refinement of these models enables Netflix to stay at the forefront of content creation in the streaming industry [10].
Zara
The fast-fashion retailer Zara uses generative AI to optimize its operations from supply chain management to customer interactions. By partnering with technology firms like Jetlore and Fit Analytics, Zara can provide personalized shopping experiences, predicting customer preferences and offering size recommendations that reduce returns and enhance satisfaction. These predictive analytics tools allow Zara to efficiently tailor their offerings to individual customers' needs [10].
These case studies highlight the versatility and impact of generative AI across various sectors, demonstrating both the opportunities and the challenges that come with its implementation. As companies continue to explore and refine these technologies, they must navigate legal, ethical, and social considerations to maximize benefits while minimizing potential risks.
Challenges and Criticisms
The usage of generative AI has sparked numerous challenges and criticisms, particularly concerning ethical considerations and societal impacts. One major ethical concern revolves around the potential biases embedded in generative AI systems. Since these technologies are trained on data sourced from the open internet, they may replicate and even amplify existing biases present in that data, leading to misinformation and disinformation, which can mislead users and propagate falsehoods [1] [11].
Another significant issue is copyright infringement. The U.S. Copyright Office has clarified that works created by generative AI are not eligible for copyright protection, as they lack the human creativity required under copyright law [12]. This has led to legal challenges, including lawsuits by entities like The New York Times, against the unauthorized use of their copyrighted materials as training data for AI models [11]. The resolution of these cases will likely have profound implications for the regulation of generative AI technologies.
The environmental impact of generative AI is also a pressing concern. These systems demand substantial energy and water resources, often exerting a disproportionate burden on socioeconomically disadvantaged regions [13]. Despite recent efforts by AI companies to secure cleaner energy sources, the transparency of environmental data remains limited, prompting legislative proposals for improved reporting [13].
Furthermore, the discussion around generative AI challenges traditional notions of originality and creativity. As AI-generated content becomes increasingly sophisticated, questions arise about what constitutes human creativity and the value of human-made versus AI-made works [14]. The emergence of AI-generated art, for example, has sparked debates over the future of human artistic expression and its distinction from machine-generated outputs [14].
Lastly, issues of plagiarism and academic integrity are heightened in the academic sphere. The misuse of AI-generated content without proper attribution can undermine the principles of academic honesty, necessitating strict guidelines and policies to ensure ethical usage [6]. These challenges underscore the need for comprehensive policies and practices to address the evolving landscape of generative AI [6].
Future Directions
The future directions for generative AI usage policies in healthcare are poised to be heavily influenced by the rapid advancements in technology and the evolving landscape of medical innovation. As generative AI continues to reshape the healthcare industry, there are several key areas that will likely require attention in the development and refinement of usage policies.
Integration with Clinical Development
The integration of generative AI into clinical development processes is expected to advance further, leveraging innovations that allow for massive data collection from diverse sources [1] [15]. Usage policies will need to address the ethical and operational implications of incorporating AI-driven methodologies in clinical trials, ensuring that data privacy and patient consent are maintained at the forefront.
Personalized Medicine and Ethical Considerations
As generative AI enables more precise and patient-centric approaches to treatment, future policies will need to focus on ethical considerations related to personalized medicine [16] [17]. This includes safeguarding genetic data, ensuring informed consent, and maintaining compliance with privacy regulations while leveraging AI to optimize individual treatment plans.
Drug Discovery and Development
Generative AI's potential to streamline drug discovery and development processes presents opportunities and challenges that will need to be addressed in future usage policies [18] [19]. These policies should consider the acceleration of drug candidate identification, optimization of molecular structures, and the management of predictive analytics related to drug interactions and adverse effects.
Real-time Decision Support and Compliance
The provision of real-time clinical decision support through generative AI will require policies that ensure adherence to legal and ethical standards [18] [20]. This involves navigating the complexities of AI-driven recommendations and maintaining transparency and accountability in AI-assisted clinical decisions.
Addressing Resource Constraints
Future policies must also account for the optimization of resources in the application of generative AI in healthcare [19] [21]. By streamlining workflows and automating routine tasks, generative AI can help overcome resource limitations, making advanced healthcare solutions more accessible and sustainable.
{
"$schema": "https://vega.github.io/schema/vega-lite/v5.json",
"description": "Generative AI Market Growth",
"data": {
"values": [
{"year": 2023, "value": 100},
{"year": 2024, "value": 150},
{"year": 2025, "value": 200},
{"year": 2026, "value": 300},
{"year": 2027, "value": 450},
{"year": 2028, "value": 600},
{"year": 2029, "value": 800},
{"year": 2030, "value": 1000},
{"year": 2031, "value": 1150},
{"year": 2032, "value": 1300}
]
},
"mark": "line",
"encoding": {
"x": {"field": "year", "type": "ordinal", "title": "Year"},
"y": {"field": "value", "type": "quantitative", "title": "Market Value (in billions)"},
"color": {"value": "#1f77b4"}
}
}
In conclusion, the responsible and ethical use of generative AI is crucial for maximizing its benefits while minimizing potential risks.

Start Your Cybersecurity Journey Today
Gain the Skills, Certifications, and Support You Need to Secure Your Future. Enroll Now and Step into a High-Demand Career !
Fusion Cyber Blogs
RECENT POSTSU.S. Coast Guard's New Cyber Rule: What Maritime Firms Must Know
The U.S. Coast Guard's new cybersecurity rule, effective July 16, 2025, mandates stricter cyber protections for maritime organizations, including risk assessments, incident response plans, and a designated Cybersecurity Officer.
Read MoreCurrent State of Federal Cybersecurity
The current state of federal cybersecurity is shaped significantly by recent initiatives and directives aimed at bolstering the United States' cyber defenses. A pivotal element in this effort is President Biden's Executive Order 14028, which underscores the urgent need to improve the nation's cybersecurity posture in response to increasingly sophisticated cyber threat
Read More