Cloud AI Technologies in Cybersecurity
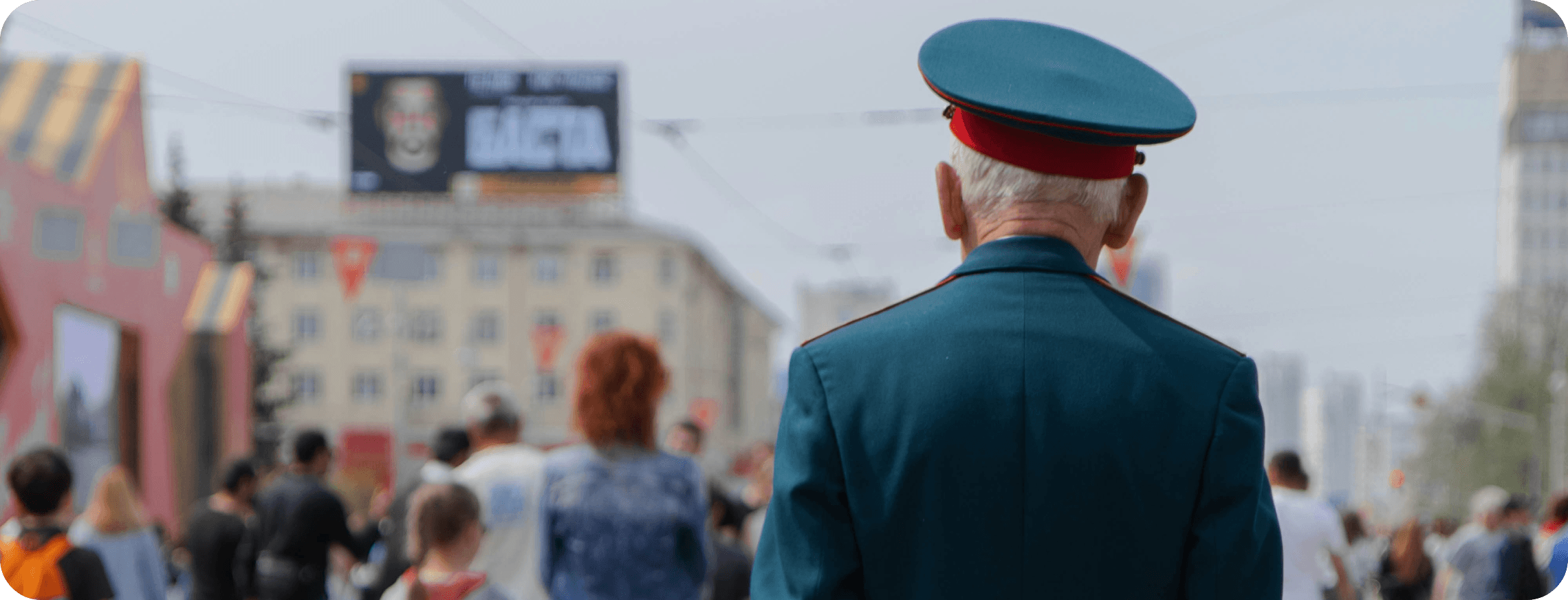
Cloud AI Technologies in Cybersecurity
The advent of cloud AI technologies has significantly transformed the cybersecurity landscape, providing organizations with advanced tools to combat increasingly sophisticated cyberthreats. By leveraging artificial intelligence (AI) in cloud environments, companies can enhance their security posture through real-time threat detection, analysis, and response capabilities [1]. AI-powered systems can process massive amounts of data to identify patterns and anomalies that may indicate a cyberthreat, enabling quicker and more accurate responses to potential security incidents [2].
Real-Time Threat Detection and Response
Cloud-based AI technologies excel at monitoring network traffic and user behavior to detect threats in real time. By analyzing data streams for unusual patterns, AI systems can identify and respond to potential security breaches much faster than traditional methods [2]. For instance, companies like Darktrace utilize machine learning algorithms within cloud environments to continuously analyze network traffic and detect anomalies that suggest security risks [1]. These systems are capable of learning from previous incidents, thus improving their threat detection and response efficiency over time.
Adaptive Security Measures
The dynamic nature of cyberthreats necessitates security measures that can adapt in real time. Cloud AI technologies provide the foundation for implementing adaptive security protocols that evolve based on the current threat landscape. Machine learning algorithms enable systems to update their defensive strategies autonomously, ensuring that security measures remain effective against new and emerging threats [1][3]. An example of such adaptive measures includes intrusion detection systems that leverage machine learning to detect and mitigate potential threats before they can exploit vulnerabilities [1].
Predictive Analysis and Risk Assessment
AI in cloud cybersecurity also enhances predictive analysis capabilities, allowing organizations to assess potential vulnerabilities and forecast emerging threats. By analyzing historical attack data and recognizing evolving cyber tactics, AI models assist in developing comprehensive risk assessments that prioritize security measures according to threat severity [1]. This proactive approach empowers organizations to strengthen their defenses against potential cyberattacks before they occur.
Reducing Human Error and Automating Tasks
AI technologies in cloud cybersecurity play a crucial role in minimizing human error by automating repetitive security tasks. Automated systems can execute tasks such as incident response and threat identification without human intervention, freeing up valuable resources and reducing the likelihood of errors associated with manual processes [2]. As AI continues to mature, its integration into cloud environments will further streamline cybersecurity operations, allowing security professionals to focus on more complex and strategic challenges.
Applications of Cloud AI in Cybersecurity
In the contemporary landscape of cybersecurity, Cloud AI has emerged as a transformative tool that significantly enhances an organization's ability to protect its digital assets. The integration of AI within cloud-based platforms offers several practical applications that bolster cybersecurity measures.
Real-Time Threat Detection
Cloud AI is pivotal in enabling real-time threat detection across digital environments. By leveraging advanced machine learning algorithms, these systems can continuously analyze network traffic and identify anomalies that may indicate potential security breaches or cyberattacks. Companies like Darktrace utilize AI-driven models to monitor network patterns, allowing for the swift identification of threats in real-time, thereby minimizing the impact on organizational operations [1].
Behavioral Analytics
Understanding user behavior is critical for identifying potential security threats. Cloud AI empowers organizations to implement behavioral analytics that track and analyze user activity patterns. AI systems learn from historical data to establish a baseline of normal user behavior, subsequently identifying deviations that could signify malicious intent or unauthorized access attempts [2]. This capability is instrumental in reducing the risk of insider threats and enhancing the security posture.
Predictive Risk Assessment
AI's predictive capabilities are leveraged to assess risks and anticipate emerging threats. By analyzing vast amounts of historical attack data, AI models can forecast potential vulnerabilities and provide comprehensive risk assessments. This approach allows organizations to prioritize their security strategies based on predicted threats, thereby fortifying their defenses [1][2].
Automated Incident Response
The automation of incident response is another significant application of Cloud AI in cybersecurity. AI systems can rapidly analyze security incidents and execute predefined response protocols, such as isolating compromised systems and applying security patches. This automation reduces the window of vulnerability and ensures a swift and coordinated reaction to potential threats [1].
Adaptive Security Measures
Cloud AI supports the deployment of adaptive security measures that evolve in real time, responding to the ever-changing threat landscape. This adaptability is crucial for maintaining robust defenses against new and evolving cyberthreats. AI-powered intrusion detection systems, for example, can continuously update their detection algorithms to address emerging vulnerabilities, providing a proactive defense mechanism [1][2].
Cyberthreat Hunting
Cloud AI facilitates proactive cyberthreat hunting by enabling security teams to actively search for potential threats within their networks. Advanced algorithms can detect subtle indicators of threats that traditional tools might miss, allowing organizations to neutralize potential risks before significant damage occurs [1]. The use of deep learning models further enhances this capability by analyzing complex data sets for signs of cyber threats [1][2].
Benefits of Cloud AI in Cybersecurity
The integration of cloud-based artificial intelligence (AI) in cybersecurity provides numerous advantages that enhance the protection of sensitive data and improve the efficiency of security operations. One significant benefit is the ability of AI to analyze vast amounts of data in real time, which enhances threat detection and response capabilities. AI algorithms are particularly adept at identifying patterns and anomalies within network traffic, allowing organizations to proactively detect potential security breaches and respond swiftly to mitigate their impact [1][2].
Additionally, AI in the cloud facilitates predictive analysis and risk assessment. By utilizing historical attack data and evolving threat patterns, AI models can foresee emerging threats and prioritize security measures accordingly [1]. This capability enables organizations to strengthen their defense mechanisms against both current and future cyberthreats, thus maintaining a robust security posture.
Another benefit is the role of AI in automating repetitive security tasks, freeing up valuable resources and reducing the likelihood of human error. Automated incident response, powered by AI, ensures that security incidents are rapidly analyzed and addressed, minimizing vulnerability windows [1]. This automation not only streamlines security operations but also allows security professionals to focus on more complex and strategic tasks.
Cloud AI also supports adaptive security measures, providing the flexibility to update security protocols in real time based on the ever-changing threat landscape [1]. This adaptability is essential for addressing zero-day threats and novel attack vectors that traditional security measures may fail to counteract.
Lastly, AI-driven behavioral analytics enhance cybersecurity by understanding user behavior patterns to identify potential insider threats and unauthorized access [1][2]. By differentiating between legitimate and malicious activities, AI can significantly reduce the risk of data breaches originating from within the organization.
Challenges and Risks
The integration of cloud computing and artificial intelligence (AI) presents significant challenges and risks that businesses must address to safeguard their operations and data. One of the primary concerns is cybersecurity, which has never been a higher priority given the elevated threats and regulatory scrutiny that accompany technological advancements [4]. The potential for data breaches is substantial, particularly for enterprises leveraging generative AI tools built on large language models (LLMs) or extensive internal datasets [5]. Such breaches can expose confidential customer information, leading to severe legal liabilities and reputational damage.
Moreover, the rapid evolution of generative AI technologies necessitates constant adaptations from developers, business users, and policymakers to manage the wide array of risks associated with AI, including privacy, cybersecurity, regulatory compliance, and intellectual property [6]. The democratization of harmful content creation through generative AI poses additional challenges, as it can exacerbate misinformation and other risks during critical periods like major elections [7].
Furthermore, the complexity of cloud service models introduces shared security responsibilities between providers and customers, which can complicate efforts to protect sensitive information [8]. Understanding these responsibilities is crucial to implementing robust security practices and ensuring data protection.
Enterprises must also navigate the regulatory landscape, as organizations like the FTC emphasize the importance of honoring privacy commitments and the severe consequences of data mismanagement [5]. This requires businesses to establish comprehensive safeguards and transparent communication regarding data practices to avoid potential legal repercussions.
To address these challenges, companies are encouraged to employ advanced AI solutions, such as Copilot for Security, to enhance their cybersecurity posture by swiftly identifying, investigating, and resolving threats [4]. By leveraging such technologies, businesses can improve their resilience against evolving cyber threats and build trust with their stakeholders [6].
Key Technologies and Tools
The intersection of cloud computing, artificial intelligence (AI), and cybersecurity has necessitated the development and deployment of various technologies and tools to ensure robust security frameworks. Among these, machine learning techniques have emerged as pivotal in fortifying cloud environments against threats. These techniques can be implemented using open-source tools to enhance the security of cloud systems effectively [9].
Machine Learning for Security
Machine learning (ML) plays a crucial role in identifying patterns and anomalies within data, which is essential for detecting potential threats in cloud systems. Various ML algorithms, such as supervised, unsupervised, and reinforcement learning, have been explored for their applicability in enhancing cybersecurity measures [9]. Anomalies in network traffic, for instance, can be detected using anomaly detection algorithms, which are a subset of machine learning techniques [9].
Generative Artificial Intelligence
Generative AI is gaining traction as a tool for cybersecurity, particularly in detecting cybercrime attacks involving ransomware viruses. This technology holds the potential to significantly improve cyber risk management systems by enhancing the detection and mitigation of threats before they can cause substantial harm [10]. The deployment of generative AI can improve systems for managing the risk of cybercrime and the loss of sensitive data, which are often archived in internal databases [10].
Federated Learning
Federated learning (FL) is an emerging machine learning approach that enhances security by not exchanging local data samples across devices, thereby protecting sensitive information [11]. This decentralized approach to model training not only mitigates privacy risks but also maintains the security and integrity of the data involved [11]. FL is considered more secure than traditional machine learning techniques because it reduces the vulnerability of data to attacks during transmission [11].
These technologies and tools collectively contribute to building a resilient infrastructure that safeguards cloud environments from an ever-evolving threat landscape. As cybersecurity continues to evolve, the integration of advanced AI methodologies and open-source tools remains imperative in addressing emerging challenges.
Case Studies
Secure AI Deployment in Financial Services
One prominent case study in the financial services industry involves a multinational bank implementing AI-driven solutions for customer service and fraud detection. To ensure secure deployment, the bank integrated security practices throughout the AI lifecycle, including secure coding, regular security testing, and vulnerability scanning [12]. The institution also established a robust architecture that incorporated zero trust principles and employed hardened containers for deploying machine learning models [13]. This approach significantly mitigated risks associated with AI-specific attack vectors, such as adversarial inputs and data poisoning [14].
Healthcare and AI Privacy Compliance
In the healthcare sector, a large hospital network adopted AI tools to enhance patient diagnostics and personalized treatment plans. A key challenge was maintaining compliance with privacy standards due to the sensitive nature of medical data. The hospital implemented a data governance framework that anonymized patient information and ensured transparent AI data usage [15]. They trained staff on AI risks and privacy issues, which helped in preventing shadow IT scenarios and maintaining data control [13]. By focusing on privacy-by-design principles, the hospital minimized data exposure risks, thereby aligning their AI initiatives with regulatory compliance [15].
Manufacturing: AI Risk Management
In the manufacturing industry, a company integrated AI technologies to optimize production processes and improve supply chain efficiency. Recognizing the potential data leakage risks from AI applications, the organization developed custom front-end interfaces for AI model interaction, which bypassed default configurations and controlled data flow [14]. They also utilized sandboxes to isolate data and created comprehensive incident response plans for potential data breaches [12]. The company prioritized secure data storage and prompt injection protection, allowing them to address data privacy concerns effectively and uphold compliance with emerging AI regulations [14].
These case studies illustrate diverse strategies for managing the cybersecurity implications of cloud-based AI deployments across various sectors. Organizations can learn from these examples to strengthen their AI security and compliance frameworks.
Future Trends
The future of cloud-AI cybersecurity is set to witness transformative trends as organizations increasingly adopt advanced technologies to fortify their defenses against ever-evolving cyber threats. One significant trend is the integration of artificial intelligence (AI) in enhancing the security of cloud environments. AI's ability to rapidly analyze vast amounts of data and identify a multitude of cyber threats—from malware attacks to anomalous behaviors indicative of phishing attempts—positions it as a pivotal component in future cybersecurity strategies [16].
Moreover, the continuous development of machine learning algorithms will allow AI systems to not only detect known threats but also predict and preempt emerging ones based on historical data and current cyber landscapes. This predictive capability will be instrumental in identifying potential network attacks, such as those initiated by malicious software and phishing emails, before they can exploit vulnerabilities [16].
Another anticipated trend is the growing emphasis on automating cybersecurity processes. As businesses increasingly rely on AI for automation, there is a concomitant need to secure these automated operations from malicious actors who might exploit AI technologies to carry out sophisticated attacks. Automation will likely involve more than just detecting threats; it will also entail implementing AI-driven responses that can dynamically adapt to new threat patterns without human intervention [16].
In addition, the rise of machine-to-machine (M2M) communication poses unique security challenges, prompting a shift towards rethinking access authorization processes in expanding computer systems. The future will likely see enhanced protocols and security measures tailored specifically to protect M2M interactions, ensuring that automated requests and data transfers are secure and authenticated [16].
The relentless advancement of AI in cybersecurity is poised to not only bolster defense mechanisms but also drive a paradigm shift in how businesses approach cyber resilience. By leveraging AI's unparalleled speed and precision in threat detection and response, companies can transform their cybersecurity posture to proactively tackle the sophisticated and organized nature of modern cyber threats [16].
In conclusion, the integration of cloud AI technologies in cybersecurity signifies a paradigm shift in how organizations defend against cyberthreats.

Start Your Cybersecurity Journey Today
Gain the Skills, Certifications, and Support You Need to Secure Your Future. Enroll Now and Step into a High-Demand Career !
Fusion Cyber Blogs
RECENT POSTSU.S. Coast Guard's New Cyber Rule: What Maritime Firms Must Know
The U.S. Coast Guard's new cybersecurity rule, effective July 16, 2025, mandates stricter cyber protections for maritime organizations, including risk assessments, incident response plans, and a designated Cybersecurity Officer.
Read MoreCurrent State of Federal Cybersecurity
The current state of federal cybersecurity is shaped significantly by recent initiatives and directives aimed at bolstering the United States' cyber defenses. A pivotal element in this effort is President Biden's Executive Order 14028, which underscores the urgent need to improve the nation's cybersecurity posture in response to increasingly sophisticated cyber threat
Read More