Generative AI in Security Operations
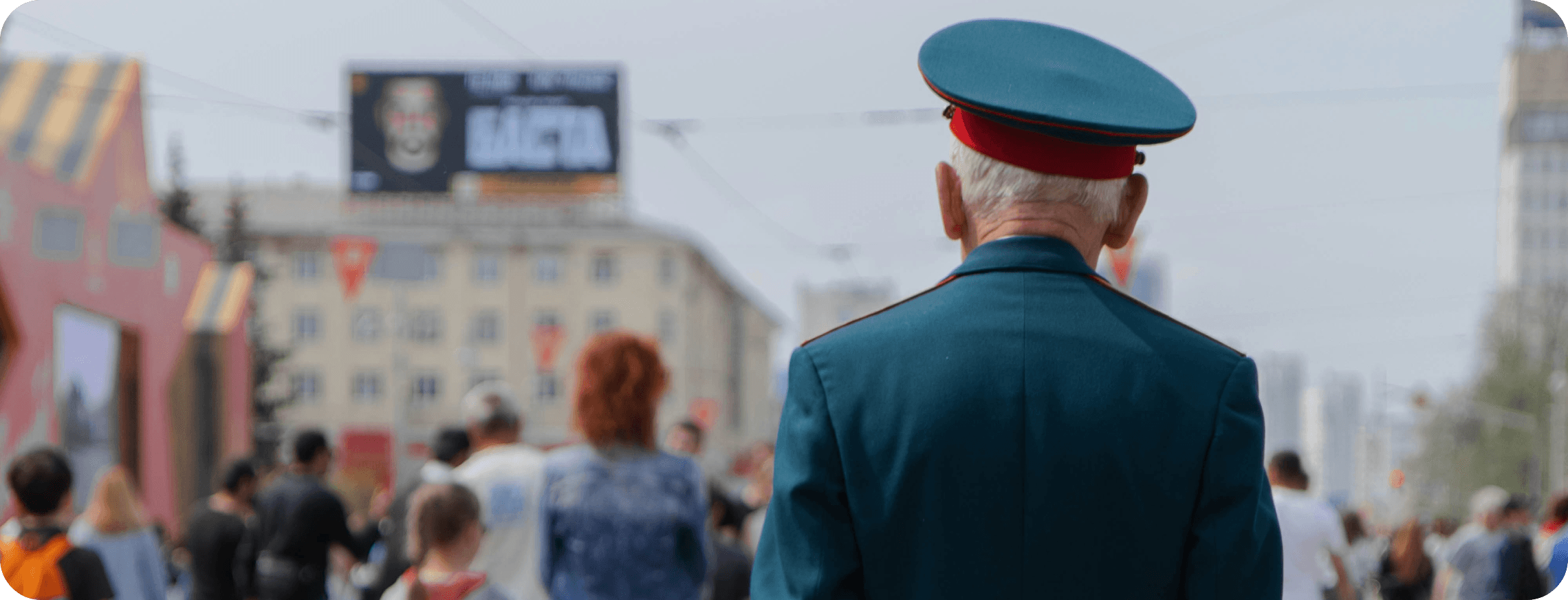
History
The evolution of security operations has been significantly influenced by advancements in automation and artificial intelligence (AI) over the decades. The journey began in the late 1980s with the development of antivirus software, which marked the initial steps toward security automation by automatically detecting and removing malware from on-premise systems[1]. The 1990s witnessed the emergence of firewalls and intrusion detection systems (IDS), which enhanced network security by automatically controlling traffic and detecting suspicious activities based on predefined rules[2].
In the early 2000s, intrusion prevention systems (IPS) advanced the capabilities of IDS by not only detecting threats but also employing decision-making processes to prevent them[3]. The mid-2000s introduced security information and event management (SIEM) technology, which facilitated real-time analysis of security alerts generated by network hardware and applications, coupled with automated alert management[4].
The integration of AI technologies into security automation became prominent in the 2010s. This period saw the incorporation of behavioral analysis and machine learning to bolster threat detection and response, addressing increasingly sophisticated cyber threats such as advanced persistent threats (APTs)[5]. Mid-2010s innovations included the advent of security orchestration, automation, and response (SOAR) platforms, which streamlined security operations by automating responses and providing insights that enabled systems to autonomously address emerging threats[6].
The 2020s have been characterized by the extension of autonomous response capabilities, allowing systems to automatically react to threats in real time. Deep learning has become more widespread, identifying complex patterns associated with threat actors and sophisticated cyber threats[6]. As generative AI becomes more integrated into security operations, it is essential for security measures to remain predictive and adaptable to effectively combat the evolving landscape of security threats[6].
Key Concepts
Generative AI (GenAI) technologies have introduced transformative changes within security operations, presenting both opportunities and challenges. At the core of these advancements is the integration of GenAI in Managed Detection and Response (MDR) services, which enhances the efficiency and efficacy of cybersecurity practices[7].
GenAI is being leveraged to automate threat detection and accelerate incident response, thereby enabling continuous monitoring of security environments[7]. Through the analysis of large volumes of data in real-time, MDR providers employ GenAI and Large Language Models (LLMs) for proactive threat detection, ultimately streamlining Security Operations Center (SOC) operations and reporting processes[7].
A crucial component of utilizing GenAI effectively in security operations is the establishment of a phased approach that incorporates security automation and orchestration. This involves identifying relevant use cases, playbooks, and workflows, which contribute to a well-defined security roadmap that maximizes the benefits of Security Orchestration, Automation, and Response (SOAR)[8].
One of the prominent applications of GenAI in security operations is in threat hunting. GenAI enhances threat-hunting capabilities by automating threat detection through the analysis of vast volumes of unstructured data from diverse sources such as news outlets, social media, and the Dark Web[7]. It further optimizes resource allocation by automating routine tasks and reducing false positives, allowing SOC Cyber Analysts to focus on more critical issues[7].
Moreover, GenAI tools improve SOC operations by providing AI-driven insights. SOC Analysts can utilize GenAI for advanced alert analysis and conduct deep threat investigations using natural language queries, leading to more informed decision-making and reduced threat investigation times[7].
GenAI also plays a significant role in automated reporting and customer communication by generating detailed incident reports and customizable alerts that keep customers informed with relevant and timely information[7]. Despite these advancements, the human expertise remains essential, particularly in understanding emerging threats and interpreting context-specific situations that GenAI may overlook[7].
Applications
Generative AI has become an integral tool in enhancing security operations by offering innovative solutions to complex cybersecurity challenges. Organizations are leveraging generative AI to bolster their defenses and improve the efficiency of their security measures. One notable application is the development of virtual security assistants, such as the one demonstrated at AWS re:Invent 2023. This assistant, built using Amazon Bedrock, Kendra, and Security Lake, provides contextual security guidance and can answer questions about IAM best practices and identify specific security findings in an environment, thereby enhancing security outcomes for teams that effectively utilize AI technologies[9].
Generative Adversarial Networks (GANs), a subset of generative AI, have gained attention for their role in network anomaly detection. GANs are used to generate new data that aids in the detection of abnormal behaviors, which are often rare and detrimental to systems. This capability is crucial in improving the effectiveness of anomaly detection methods, making GANs valuable tools in cybersecurity[10][11].
Moreover, generative AI is being employed to predict and prevent incidents before they occur, thereby contributing to digital resilience. By harnessing AI and machine learning, organizations can anticipate potential threats and mitigate risks, reducing downtime and enhancing their overall security posture[12].
In the context of ethics and compliance technology, companies like GAN Integrity are using AI to streamline vulnerability management processes. By employing advanced tools like reachability analysis, they can filter out false alarms and focus on genuine threats, improving operational efficiency without compromising security[13].
Benefits
The integration of Generative AI in security operations centers (SOCs) brings a myriad of benefits, particularly in enhancing operational efficiency and strategic focus. One significant advantage is the automation of repetitive and low-level tasks, which allows security teams to allocate their time and resources to more critical initiatives, such as threat hunting and improving the overall security posture[14]. This shift not only maximizes the productivity of security personnel but also optimizes incident response times, which is crucial when every second counts[14].
Additionally, the deployment of automation in security operations facilitates rapid processing of incidents. For instance, through Generative AI, SOCs can efficiently respond to phishing attacks, conduct malware investigations, and address zero-day threats, thereby minimizing potential damage and ensuring swift action[14]. The streamlined processes also extend to managing threat intelligence feeds and provisioning remote user access, further reinforcing the security infrastructure's robustness and scalability[14].
By enabling these advanced capabilities, Generative AI significantly enhances the operational efficiencies within SOCs. This allows security teams to not only manage existing threats effectively but also to proactively anticipate and mitigate future security challenges, thereby strengthening the organization's overall security posture in an increasingly complex threat landscape[14].
Challenges
The integration of generative AI into security operations presents several formidable challenges. One significant issue is the increasing sophistication of cyber threats, where advanced persistent threats and malware have the potential to compromise entire networks[15]. Organizations must leverage all available resources, including data, to effectively combat these evolving security risks[16].
In the realm of content moderation and trust and safety, the democratization of harmful content creation driven by generative AI, such as deepfakes, poses a significant threat[17]. Deepfakes and other forms of manipulated media can be weaponized for identity theft, spreading misinformation, political manipulation, and defamation[18]. These issues highlight the need for robust detection and prevention measures, such as the use of Generative Adversarial Networks (GANs), to safeguard digital environments from maliciously altered media[19].
Furthermore, the implementation of AI systems must balance accuracy and privacy. An over-reliance on automated detection can lead to false positives, allowing harmful content to bypass safeguards or wrongfully flagging benign content[19]. This underscores the necessity for transparent AI systems that ensure user data protection while effectively identifying and mitigating threats.
Additionally, as AI technologies advance, compliance with evolving regulations becomes a critical challenge. Organizations must navigate complex legal landscapes and adhere to various frameworks to maintain compliance and uphold trust with stakeholders[20]. This requires continuous monitoring and adaptation to new regulatory requirements, which can be resource-intensive and necessitate sophisticated compliance automation solutions[18].
Technologies and Tools
The rise of synthetic media and the threats posed by manipulated content such as deepfakes have necessitated the development of advanced technologies to bolster digital security operations. Among these, Generative Adversarial Networks (GANs) stand out as a promising tool in the fight against media manipulation. Initially introduced by Ian Goodfellow and his colleagues in 2014, GANs comprise two neural networks—the generator and the discriminator—that work in tandem through a competitive process to produce and verify synthetic data[18][19].
Generative Adversarial Networks (GANs)
Structure and Functionality
GANs consist of a generator that creates synthetic media resembling real data, and a discriminator that evaluates the authenticity of this generated content[18]. Through continuous training, these networks refine their abilities: the discriminator becomes more adept at identifying fakes, while the generator enhances its capability to produce realistic content. This adversarial learning process results in sophisticated models that can both recognize and generate high-fidelity synthetic media[19].
Application in Detection
GANs serve as a powerful mechanism for identifying and mitigating deepfakes and other forms of altered media. By training on datasets that include both genuine and fabricated content, GANs can discern subtle irregularities indicative of manipulation[19]. This technology enables high accuracy in detecting variations in elements such as audio artifacts, lighting conditions, and facial expressions, making it a vital component in modern security operations[18].
Content Moderation
In the context of social media and digital platforms, GANs offer significant advantages for content moderation. They are capable of real-time analysis and automatic flagging of suspect content, thereby preventing the rapid spread of harmful media[18]. This capability is essential in maintaining the integrity of online information and protecting individuals from potential reputational damage and identity theft.
Additional Security Tools
While GANs play a critical role, other AI-powered tools complement these efforts by focusing on risk management and compliance. For instance, platforms like Akitra leverage AI for compliance automation, offering solutions to prevent data breaches and ensure adherence to various security frameworks such as SOC 2, HIPAA, and GDPR[18]. These platforms integrate functionalities such as vulnerability assessments, pen testing, and automated questionnaire responses, providing comprehensive security solutions tailored to the needs of fast-growing companies.
The integration of GANs and other AI-based technologies into security operations underscores the evolving landscape of digital protection, offering robust defenses against the challenges posed by synthetic media. Through continued advancements and ethical deployment, these tools promise to uphold digital trust and authenticity in an increasingly complex digital environment[19].
Case Studies
Amazon Web Services
In a recent demonstration at AWS re:Invent 2023, Amazon Web Services showcased a virtual security assistant developed using Amazon Bedrock, Kendra, and Security Lake. This assistant is designed to enhance security outcomes by leveraging generative AI to provide contextual security guidance. The demonstration highlighted the architecture, implementation, and key considerations for safely and effectively using generative AI in security operations[9]. The virtual assistant was shown to answer queries about Identity and Access Management (IAM) best practices and identify specific security findings within an environment. This approach emphasizes the potential of generative AI to support, rather than replace, security experts, suggesting that teams utilizing these technologies can significantly improve their security operations[9].
Future Trends
As the landscape of cybersecurity continues to evolve, future trends in generative AI for security operations are emerging with a focus on enhanced detection and prevention mechanisms. Security Operations Centers (SOC) are adapting by integrating advanced AI technologies to stay ahead of sophisticated threats, driven by the continuous rise in digital transformations and increasing threat vectors[21][22]. The need for SOC transformation is becoming urgent due to the proliferation of remote access and the lucrative nature of ransomware attacks, necessitating a reevaluation of strategies and capabilities[22].
Generative Adversarial Networks (GANs) are anticipated to play a pivotal role in combating deepfakes and other forms of manipulated media, which pose significant risks such as misinformation, identity theft, and political manipulation[18]. The evolution of GANs involves using adversarial training techniques to improve detection accuracy, allowing these models to adapt and recognize new forms of altered content effectively[18][19]. This advancement ensures that GANs can continue to identify subtle irregularities in synthetic media, thereby safeguarding digital integrity[19].
Furthermore, the application of GANs in content moderation is expected to expand, providing scalable solutions for large platforms challenged by the widespread dissemination of falsified content[19]. The implementation of GAN-based detection systems will require a balanced approach to ensure both accuracy and privacy, protecting user data while minimizing false positives[18].
As security operations integrate AI-driven compliance and risk management platforms, such as those offered by Akitra, organizations will enhance their ability to prevent sensitive data breaches and ensure adherence to multiple regulatory frameworks[18]. This comprehensive approach to security and compliance will be critical as SOCs aim to streamline processes and achieve cost-effective certifications[18].
Criticisms
Despite the promising applications of Generative Adversarial Networks (GANs) in enhancing security operations, several criticisms and challenges have emerged regarding their use. One primary concern is the dual-use nature of GANs, which can generate highly realistic synthetic media that is indistinguishable from genuine content, thus facilitating the creation of deepfakes and other forms of manipulated media. This poses significant risks, including identity theft, misinformation, and political manipulation, as GANs can be exploited by malicious actors to produce fraudulent content that undermines digital trust and authenticity[18][19].
Moreover, the implementation of GAN-based detection systems in security operations raises concerns about privacy and accuracy. Striking the right balance between these elements is crucial, as over-reliance on automated methods can lead to false positives, while insufficient reliance may allow harmful content to evade detection. Ensuring that these technologies operate transparently and protect user data is essential to maintaining public confidence and trust[19].
Furthermore, the development and deployment of GANs require significant computational resources and expertise, which may not be readily accessible to all organizations. This disparity can create challenges in adopting GAN-based solutions across diverse sectors, potentially leaving some entities more vulnerable to advanced cyber threats[18].
Lastly, ethical considerations arise with the deployment of GANs, as the technology can inadvertently perpetuate biases present in the training data. This necessitates careful curation and preprocessing of datasets to ensure that GANs do not reinforce or amplify existing prejudices, which could have unintended social and ethical implications[19].
In conclusion, generative AI is revolutionizing security operations by enhancing threat detection, response, and overall cybersecurity resilience.

Start Your Cybersecurity Journey Today
Gain the Skills, Certifications, and Support You Need to Secure Your Future. Enroll Now and Step into a High-Demand Career !
Fusion Cyber Blogs
RECENT POSTSU.S. Coast Guard's New Cyber Rule: What Maritime Firms Must Know
The U.S. Coast Guard's new cybersecurity rule, effective July 16, 2025, mandates stricter cyber protections for maritime organizations, including risk assessments, incident response plans, and a designated Cybersecurity Officer.
Read MoreCurrent State of Federal Cybersecurity
The current state of federal cybersecurity is shaped significantly by recent initiatives and directives aimed at bolstering the United States' cyber defenses. A pivotal element in this effort is President Biden's Executive Order 14028, which underscores the urgent need to improve the nation's cybersecurity posture in response to increasingly sophisticated cyber threat
Read More